UCI, other researchers develop deep-learning technique to ID at-risk anatomy in CT scans
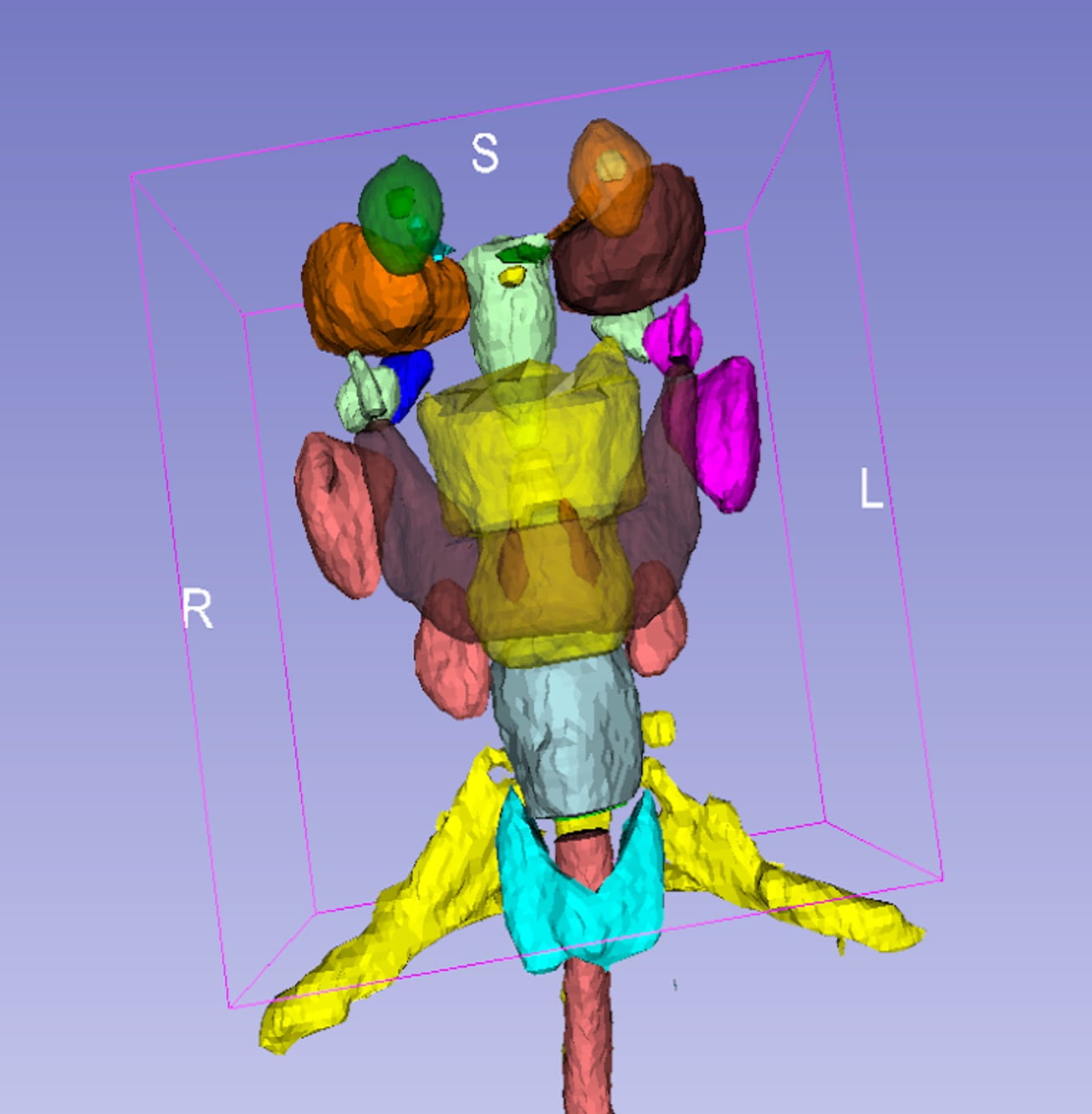
Radiation therapy is one of the most widely used cancer treatments, but a drawback of the procedure is that it can cause collateral damage to healthy tissue in proximity to cancerous growths. Identifying organs at risk via CT scans is a difficult and labor-intensive process, but UCI computer scientists and researchers from other institutions have developed an automated technique to perform this function using a deep-learning algorithm. Their work was published recently in Nature Machine Intelligence. “Using our model, it’s possible to delineate an entire scan in a few seconds, a task that would take a human expert over half an hour,” said co-author Xiaohui Xie, UCI professor of computer science. “On a data set of 100 CT scans, our deep-learning method achieved an average similarity coefficient of more than 78 percent, a significant improvement over analyses done by radiation oncologists.” The researchers focused on the head and neck for their study because of the complex anatomical structures and dense distribution of organs in this part of the body. Also, unintended irradiation to sensitive tissue in this area can lead to adverse side effects such as difficulty in opening the mouth, deterioration of vision and hearing, and cognitive impairment. Xie said the success of his team’s approach can be attributed to the model’s two-stage design. First the system identifies regions containing vital organs, and then it extracts image features from these focal areas. “Our deep-learning neural network greatly enhances the ability to delineate anatomies even with low-contrast CT scans,” Xie said. “And the setup is more computationally efficient than other methods, enabling it to be done with more standard levels of graphics processing unit memory. This means the technique can be deployed more readily in actual clinics.” His collaborators were from China’s Shanghai Jiao Tong University School of Medicine and DeepVoxel Inc. of Costa Mesa.